Dynafor présent à la conférence ForestSAT 2022
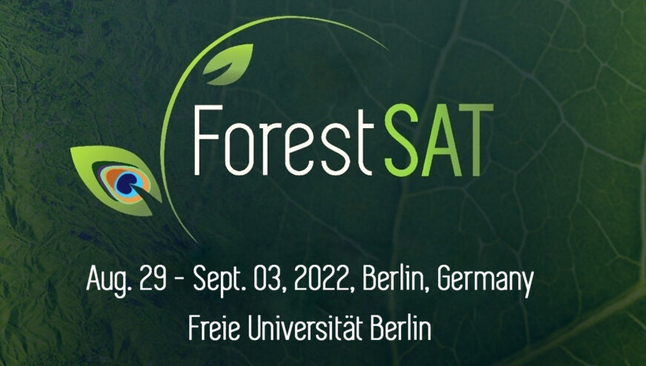
Une communication orale et un poster illustrant les travaux en télédétection forestière de l’unité DYNAFOR ont été présentés à la conférence internationale ForestSAT 2022 à Berlin (et en ligne) du 29 août au 3 septembre 2022.
Pour en savoir plus, voilà les résumés:
Jean-Philippe Denux, Jean-Marie Savoie, Véronique Chéret : Detecting standing dead trees in mountain fir forest, an indicator for health status and maturity (communication orale)
Dead wood amount can be related to forest stand wildlife habitat and biodiversity as well as diseases and pest’s damages. We aim to map standing dead trees density as an indicator of environmental quality of fir forests. In managed forest stands it could be used as a health status indicator, in old growth unmanaged forest it is a maturity indicator. Our study area covers 1000 km² in Pyrénées mountain range, at the border between France and Spain. Fir and other coniferous forests span between 600 m to 2000 m covering an area of about 25000 ha. Very high resolution (0.2m) aerial photographs were acquired in 2016 and 2019. We managed a mosaic of 25 tiles of 5x5 km, 3 bands image. Coniferous forest areas were mapped with National Forest Inventory data. A single shot detector (SSD) deep learning model was used. This model is based on ResNet-34 convolutional neural network (CNN) with 6 supplementary layers used to extract rectangular objects at various scales. To train the model 620 dead trees were localized visually on 2018 aerial photographs. For each dead tree, a 256x256 pixels image chip was extracted. Data augmentation processes were applied, for each image chip, we performed 90 degrees, 180 degrees and 270 degrees clockwise rotations and a 52 pixels translation. The model was trained with 2500 epochs, with 66% of training data. Detected objects are square or rectangular (shape ratio 1/1 or 1/1.5), with less than 10% overlay. Remaining 33% training data were used for accuracy assessment calculating average precision score. Results of the model shows an average precision score of 0.845. The centroid of each dead tree was mapped for years 2016 and 2018. Using a moving window of 100x100m to count the number of standing dead tree per hectare and to map the result at 20 m resolution. The results were analyzed using altitude ranges. In fir forest stands, standing dead trees density increases when altitude decreases, the average dead tree per hectare rises from 0.7 for the highest stands (higher than 1700 m) to 1.4 for the stands at low altitude (from 750 m to 1250 m). 1900 hectares of old growth forest have been surveyed and mapped in our study area, we compared dead tree density inside and outside these forests. In these mature forests, standing dead trees density is higher, for the same altitude classes, it rises from 2.0 to 3.3. With this dataset we observed that standing dead trees densities systematically increased from 2016 to 2019.
Véronique Chéret1, Jean-Philippe Denux1, Michel Goulard1, Michel Chartier2, Julie Vigouroux1 (1 Univ. Toulouse, INRAE, UMR DYNAFOR, Castanet-Tolosan, France; 2 CNPF-IDF, Orléans, France): Monitoring health status of chestnut forest stand using Sentinel-2 images (poster)
In France, chestnut is one of the forest species the most frequently impacted by forest decline. End-users’ needs are about the damage’s extent and monitoring of the health status of these chestnut stands. The model proposed to predict chestnuts health status is based on Sentinel-2 images. We aim to test the model capability to be applied on various territories and to be updated without new acquisitions of costly field reference data. The study area is the Nouvelle-Aquitaine region. Chestnut forest covers about 100 000 hectares, 4 Sentinel-2 tiles acquired in 2020 and 2021 were processed. The proposed model aims to discriminate healthy chestnut forest stands from the declining ones with 4 levels of withering intensity. Field surveys were based on 40 by 40 m plots (4 pixels at 20m resolution), where the health status of 72 chestnut trees was diagnosed using DEPERIS method. In 2020, 103 plots were surveyed for model calibration and development. In 2021 174 plots were surveyed for validation. The model was applied, without new calibration, on 2 Sentinel-2 tiles covering another chestnut forest area in Ile-de-France region. The first step of the model design is the selection of the most efficient variables to establish the health status of the chestnut stand among 10 spectral bands, 30 vegetation indices and 5 biophysical parameters. The sequential features selection approach is based on random forest classifier, it showed that the maximum precision can be reached with 10 variables. Secondly, the model is based on an ordered polytomous regression. This regression allows to estimate, with the 10 selected variables, the probability for each of the 4 levels of withering intensity observed. A synthesis map presents for each pixel the level with the highest probability. In the Nouvelle-Aquitaine region, for 2020, results showed a kappa index of agreement equal to 0.62, an overall accuracy equal to 0.74. For the same region, for 2021, the coefficient of correlation between the field survey and the model levels is 0.86. In the Ile-de-France region, for 2020, the model result was compared to a supervised classification of the levels of withering intensity. Even if the two nomenclatures are not identical, most of the patches of forest decline are identified by both approaches, 67% of the pixels of the highest level of withering are alike.
Comments